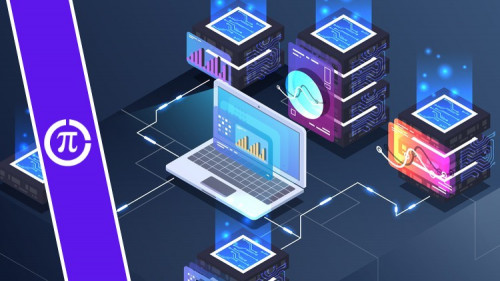
Udemy – Create & Deploy Data Science – Deep Learning Web Apps 2021
English | Tutorial | Size: 1.21 GB
Learn development & deployment of machine learning and deep learning application projects with python on heruko
Deployment of machine learning models means operationalizing your trained model to fulfill its intended business use case. If your model detects spam emails, operationalizing this model means integrating it into your company’s email workflow-seamlessly. So, the next time you receive spam emails, it’ll be automatically categorized as such. This step is also known as putting models into production.
Machine learning models are deployed when they have been successful in the development stage-where the accuracy is considered acceptable on a dataset not used for development (also known as validation data). Also, the known faults of the model should be clearly documented before deployment.
Even if your spam detection model has a 98% accuracy it doesn’t mean it’s perfect. There will always be some rough edges and that information needs to be clearly documented for future improvement. For example, emails with the words “save the date” in the subject line may always result in a spam prediction-even if it isn’t. While this is not ideal, deployment with some of these known faults is not necessarily a deal breaker as long as you’re able to improve its performance over time.
Models can integrate into applications in several ways. One way is to have the model run as a separate cloud service. Applications that need to use the model can access it over a network. Another way is to have the model tightly integrated into the application itself. In this case, it will share a lot of the same computing resources.
How the model integrates within your business systems requires careful planning. This should ideally happen before any development begins. The setup of the problem you are trying to solve and constraints under which models need to operate will dictate the best deployment strategy.
For example, in detecting fraudulent credit card transactions, we need immediate confirmation on the legitimacy of a transaction. You can’t have a model generate a prediction sometime today only to be available tomorrow. With such a time constraint, the model needs to be tightly integrated into the credit card processing application and be able to instantaneously deliver predictions. If over a network, it should incur very minimal network latency.
For some applications, time is not of the essence. So we can wait for a certain amount of data to “pile up” before the machine learning model is run on that data. This is referred to as batch processing. For example, the recommendations you see from a shopping outlet may stay the same for a day or two. This is because the recommendations are only periodically “refreshed.” Even if the machine learning models are sluggish, it doesn’t have a big impact as long the recommendations are refreshed within the expected time range.
RAPIDGATOR
rapidgator.net/file/668be48ae09ac64f0d3e7da2a5c18cf7/Udemy_-_Create_&_Deploy_Data_ScienceDeep_Learning_Web_Apps_2021.part1.rar.html
rapidgator.net/file/02fb5fc5ff4598350221a315cd37d97b/Udemy_-_Create_&_Deploy_Data_ScienceDeep_Learning_Web_Apps_2021.part2.rar.html
NITROFLARE
nitroflare.com/view/FCC7FFF2CD7C0B3/Udemy_-_Create_%26_Deploy_Data_ScienceDeep_Learning_Web_Apps_2021.part1.rar
nitroflare.com/view/FE5220D775E6D1A/Udemy_-_Create_%26_Deploy_Data_ScienceDeep_Learning_Web_Apps_2021.part2.rar