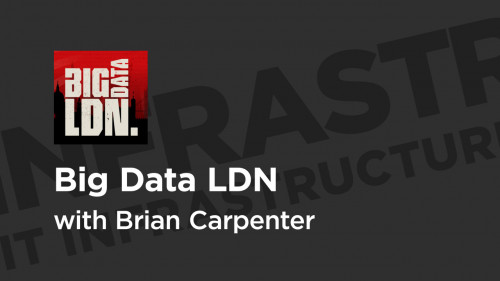
Pluralsight – Modernise Your It Infrastructure For Ai-NOLEDGE
English | Size: 80.45 MB
Category: Tutorial
Notes: Big Data LDN 2019 | Modernise Your IT Infrastructure for AI | Brian Carpenter
In this session, learn about the latest best practices for designing end-to-end AI platforms for your data science teams. You can seamlessly fit AI projects into a unified analytics plan with the right infrastructure built for scaling Brian Carpenter will map out how Pure Storage can support your overall AI strategy by walking through an example AI data hub microservices deployment